The Role of IoT, Edge Computing and AI in Industry Evolution
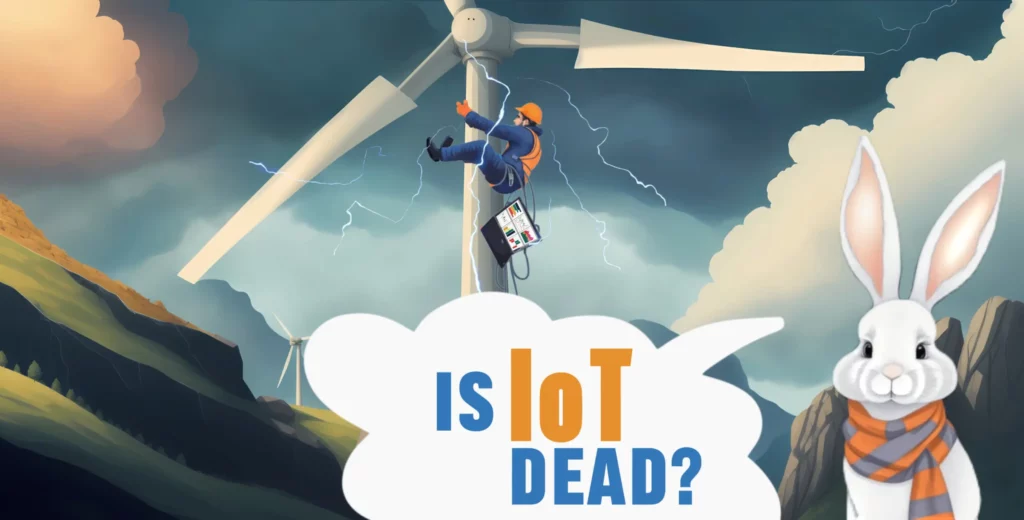
In this article, we delve into the fusion of IoT, edge computing and AI, highlighting their collaborative capabilities across multiple industries. From real-time analytics to predictive maintenance, these technologies are moving beyond mere buzzwords to serve as enablers of innovation and efficiency.
The journey started with the initial hype around IoT about a decade ago, followed by the emergence of Edge Computing, and currently the spotlight is on AI, specifically Machine Learning (ML), which reigns as the most discussed area within the AI landscape.
In recent years, IoT, edge computing and AI have garnered a lot of attention, with each making a unique contribution to the technology landscape. Let’s begin our journey by examining the current state of IoT.
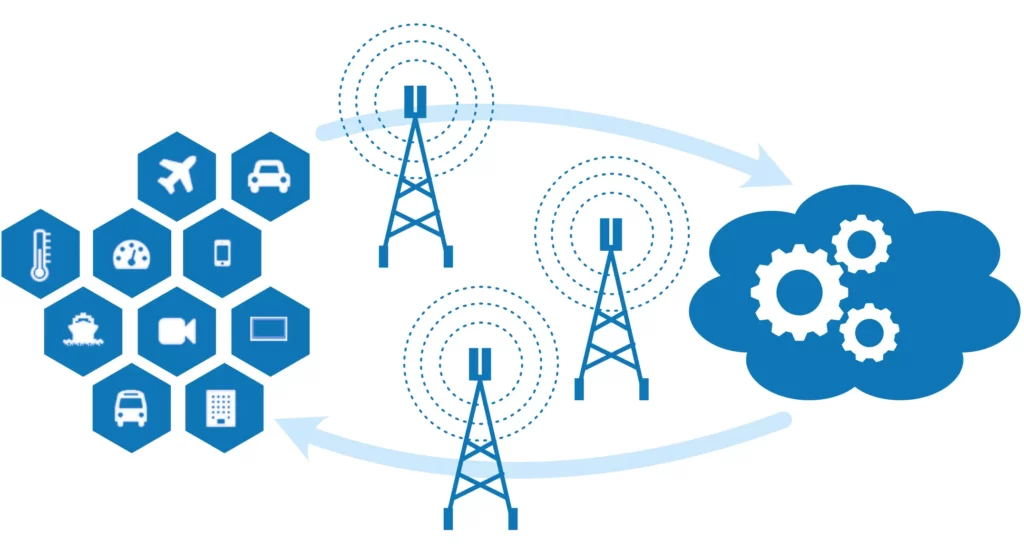
Is IoT Losing Steam?
Many of the hundreds of IoT platforms built over the past decade have faded into obscurity, leaving only a handful standing. Notable survivors include the IoT platforms of major cloud providers such as AWS and Azure, while Google said goodbye to its IoT platform in 2023. However, this isn’t a sign of IoT’s demise; rather, the underlying technologies have matured into commodities that have firmly established their presence. The industry has realized that an ‘IoT platform’ alone is not enough to overcome business challenges. Instead, the focus has shifted to developing industry-specific solutions that address real business needs and leverage mainstream IoT technologies.
Driving IoT Applications
Consider this: almost every new car sold in the last five years functions as an IoT device, equipped with a built-in IoT gateway known as a ‘telematics control unit’ (TCU) or ‘smart antenna’. These TCUs, which are essentially ARM-based computers running Linux, connect to cloud infrastructure via mobile internet (now 5G) using protocols such as MQTT, while also connecting to other car systems via CAN or Ethernet. Applications range from transmitting diagnostic data to the manufacturer’s cloud platform for analysis, to enabling interactions with mobile apps for tasks such as door lock/unlock and engine start/stop. From a cost perspective, efficient frameworks such as macchina.io EDGE are ideal, resulting in millions of cars already equipped with TCUs running macchina.io EDGE.
Similarly, low-cost industrial IoT gateways are extending the capabilities of industrial automation systems, sometimes even replacing more expensive industrial PCs. In certain scenarios, these gateways, powered by frameworks such as macchina.io EDGE, can control equipment, potentially replacing traditional PLCs. However, this approach is limited to applications that do not require strict safety or real-time constraints. In addition, IoT gateways can act as flexible protocol translators, facilitating the integration of equipment into existing infrastructures. For example, a manufacturer of fuel cell-based power generators used a low-cost IoT gateway as a central communications hub, connecting to the system’s PLC and various sensors/actuators, and supporting multiple protocols, including MQTT, REST, SNMP, as well as industry-specific standards such as DNP3 or IEC 61850 that are used in electrical power grid infrastructure. In addition, the gateway implements a web-based user interface to the system, which can also be accessed remotely in a secure way thanks to macchina.io REMOTE.
The Future Outlook for IoT
Although the initial IoT buzz has died down, IoT has gained a foothold and is here to stay. The focus has shifted from generic IoT platforms to bespoke IoT applications, often assembled using off-the-shelf components, rather than relying on a single platform. In the midst of the ongoing AI and ML surge, IoT is playing a crucial role in collecting and providing the data that fuels AI algorithms, effectively giving AI a purpose.
The Rise of Edge Computing
In response to the cloud computing boom of the late 2010s, edge computing emerged as a counterculture, advocating the decentralization of certain applications to local systems at the edge of the network, closer to data sources. Edge computing typically involves physical elements, as it deals with data from sensors or devices, or requires physical actions via actuators. Whether in autonomous driving systems that rely on sensor data or industrial setups powered by IoT devices, edge computing shines in scenarios that require resilience to network failures or demand low latency.
While edge computing isn’t a new concept – industrial vision systems have been operating near production lines for years – modern iterations integrate system management capabilities, enabling automated provisioning and orchestration of edge computing workloads from a centralised platform. Docker containers form the backbone of these platforms, enabling centralised management and deployment across diverse edge computing devices ranging from embedded systems and IoT gateways to industrial PCs and rack-mounted servers.
IoT and edge computing are often complementary, with IoT systems providing data or controlling actuators based on the results of calculations. Entire IoT systems can even run on edge devices, such as scenarios where macchina.io EDGE in a Docker container collects sensor data and sends it to a local InfluxDB database, which is then visualised using Grafana. In addition, local data analysis with pre-trained neural networks is possible, as inference tasks require less computing power compared to training, often requiring the use of AI accelerator hardware such as NVIDIA Jetson in edge systems.
Artificial intelligence and machine learning
Finally, we delve into the field of Artificial Intelligence (AI) and Machine Learning (ML), the current technological darlings. AI encompasses a wide range of algorithms and technologies, including neural networks, deep learning, rule-based systems, and Bayesian inference, among others. ML, a subset of AI, enables systems to learn and improve by analysing large data sets, often using models such as artificial neural networks and deep learning, which are characterised by numerous layers of neurons.
While generative AI is attracting attention, particularly text and image generators such as GPT and Stable Diffusion, AI applications that analyse sensor data are of paramount importance. This is where IoT systems, or systems built on IoT technologies, play a critical role in feeding AI with data. Typically, machine learning systems run on edge systems, while the resource-intensive training takes place in the cloud. The trained model parameters are then transferred to edge systems for execution (inference), which often requires significant computing resources, hence the prevalence of AI accelerator hardware in edge setups.
Conclusion
In many applications involving real-world data, the convergence of IoT, edge computing and AI provides a powerful combination. IoT systems provide the data, edge computing infrastructure decentralizes computation close to the data sources, and AI provides the algorithms for data analysis.